Luma Dream Machine vs Kling - A brief comparsion using APIs

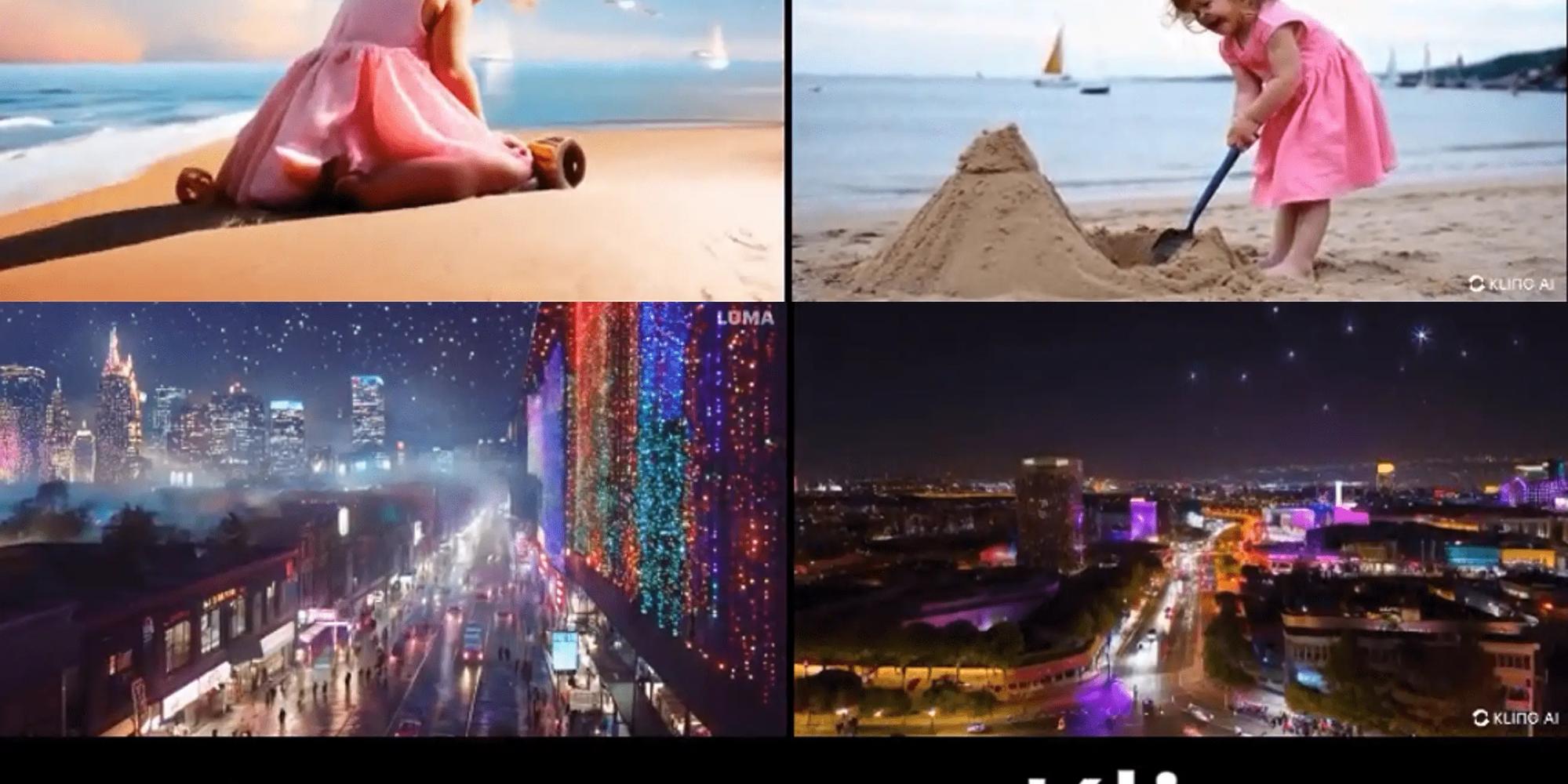

Introduction
As OpenAI's text-to-video model Sora rapidly gained popularity after the release of its several high-definition previews on Feb 15th 2024, the AI ecosystem was eagerly waiting for months for its launch to experiment with this newest text-to-video technology but they were of no avail. However, on June 12th, Luma's Dream Machine was launched for public use. And two days prior, on June 10th, the Chinese short video platform Kuaishou released their own text-to-video model Kling. Both product launches were able to make significant dents in the text-to-video generation space, with developers, tech enthusiast and content creator quickly utilizing the tools as part of their workflow.
Thus, PiAPI as the API provider for both Luma's Dream Machine and Kling, would like to briefly compare the two models and share as a reference for our users.
Evaluation Framework
Regarding the evaluation framework used for this comparison, we'd like to adopt the same framework as we had used in our previous blog comparing Luma Dream Machines 1.0 vs 1.5, namely:
- Prompt Adherence
- Text Adherence
- Video Realism
- Artifacts
For what each of these aspects mean and how they could be rated, feel free to read our previous blog for more detail.
Same Prompt Comparison
To effectively compare the outputs of these two models, we have decided to use the same prompt for both models. The prompts are submitted to Dream Machine and Kling using our Dream Machine API (or Luma API), and our Kling API and the returned results are provided along with their analysis, as shown below.

Prompt Adherence
Both models shows important elements described in the prompt: an afternoon, sunlight, sandy beach, ocean, the litter girl with blonde hair and pink dress, and the boats in the far distance.
The Luma model was able to get the seagull and sandal details well; whereas the Kling model was able to get the "toe buried in the sand" part well.
The Luma model wasn't able to get shovel and the sand castle details where Kling depicted them well.
Video Realism
The Luma Model offered a slightly different visual effect, perhaps a bit too ideal and too unblemished. Kling's output on the other hand resembles the real-world more closely, with natural lighting and proportions.
Video Resolution
The Luma Model's resolution is high, with details like the texture of the hair, clothing, and the sparkle of the sea clearly visible, but the overall effect appears somewhat smooth due to the artistic processing. Kling's output also has high resolution, with details such as the sand, waves, and the girl’s hair strands appearing clear and natural, consistent with real-world resolution.
Artifacts
The depiction of the little girl's knees and legs are quite off in Luma's output, perhaps it is due to there is very little training data on that particular w-sitting position. And since Kling's output has the little girl standing up, one could make the assumption that Kling would have a similarly difficult time portraying the sitting posture accurately and realistically.
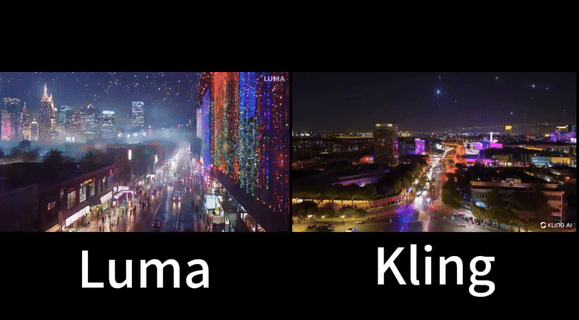
Prompt Adherence
Both models captured most of the prompt descriptions: the night city, the neon lights, the overviewing camera angle, busy streets with people and cars. However, both models missed the slowly descending camera view part, perhaps it was due to the usage of the word "slowly".
Video Realism
Both videos showed a decent level of realism with the night lighting. The city skyline portrayed in the Luma video seems a bit whimiscal.
Video Resolution
Both videos had a relatively good quality of resolution.
Artifacts
The video from Luma shows some unnatural transitions between the lights in the background and those on the streets, particularly around the edges of buildings, where there is slight distortion and blurring. For Kling's video, the way that the vehicles shrinks in size and rapidly disappears as they approach the end of the street look very unnatural.
Conclusion
So above are the two examples of both text-to-video generation model processing complex, dynamic scenes with multiple details. It is up to readers like you to decide which model fared better, our team at PiAPI simply proposes a framework to evaluate the outputs and provide real test outputs for you :)
We hope you found this comparison blog useful! If you are interested, please also check out PiAPI for our other generative AI APIs!